Okay, so I’ve been trying to get my head around this “Monfils prediction” thing. It’s been a bit of a rollercoaster, I’m not gonna lie.
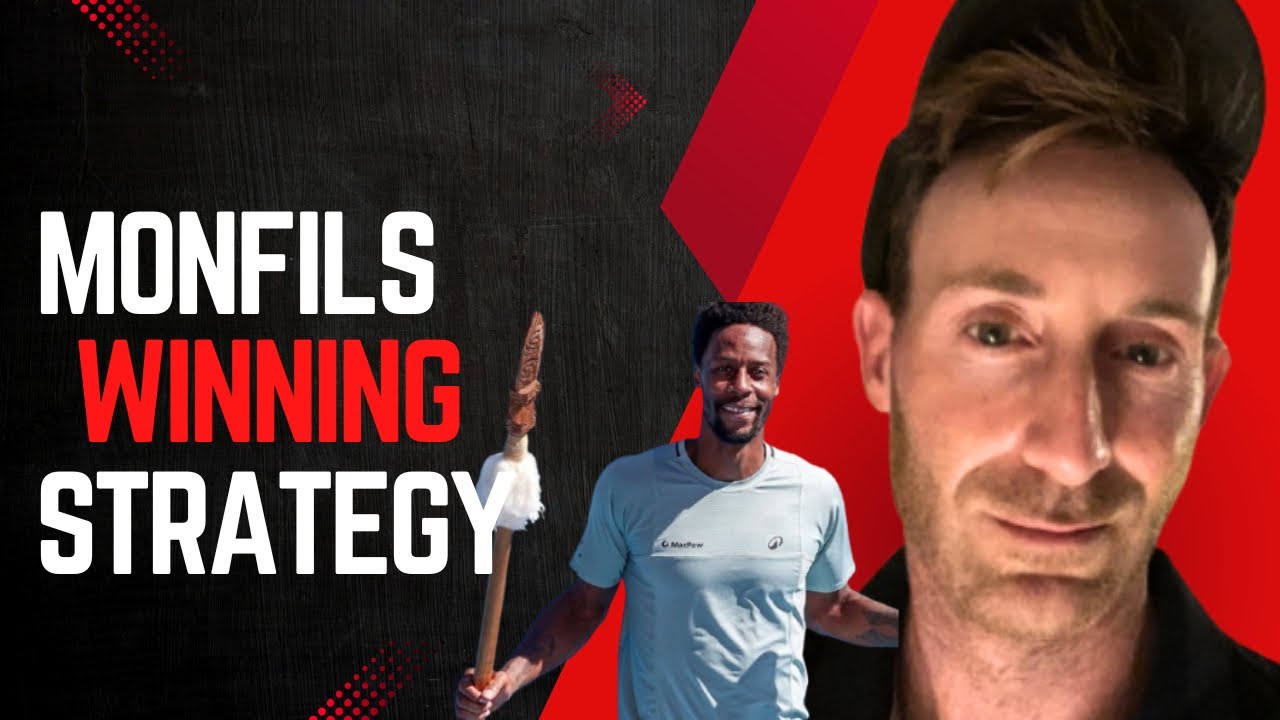
Diving In Headfirst
First, I started looking everywhere for information. You know, just Googling stuff like “Monfils prediction methods,” “Monfils stats analysis,” and things like that. I was basically throwing everything at the wall to see what stuck.
Getting My Hands Dirty
I started by collecting data, a lot of it. I grabbed match results, player stats, even things like weather conditions (yeah, I went there). I dumped it all into a big spreadsheet. It looked pretty messy at first, but hey, that’s part of the process, right?
- Gather match data: Win/loss records, scores, opponents.
- Find Player statistics: Aces, double faults, winning percentage, etc.
- Even got surface preference data: Clay, grass, hard court performances.
Experimenting Like Crazy
I started trying different things. I messed around simple formulas at first, just calculating win percentages and stuff. Then I tried some basic prediction models.
I tinkered, I tweaked, I broke things, and then I fixed them (sometimes). It was all about finding what worked and what didn’t.
Seeing the (Faint) Light
After a lot of trial and error, something started to emerge. I found certain factors that seems have higher correlations, such as history records, winning percentage and even the surface of the court!.
Still a Work in Progress
I’m not gonna pretend I’ve cracked the code or anything. It’s definitely still a work in progress. But I’ve got something that’s showing promise, and that’s pretty exciting. I’m going to keep refining it, adding more data, and see where it goes. It’s a journey, and I’m here for the ride!